Navigating Abuse in the Age of AI - Taylor Swift's Deep Fake Case Study
Unraveling the fallout from Taylor Swift's deep fake saga, a stark reminder of AI's dark side. Dive into the implications as tech giants react, signaling a new era of AI regulation and the urgent need for innovative safeguards.
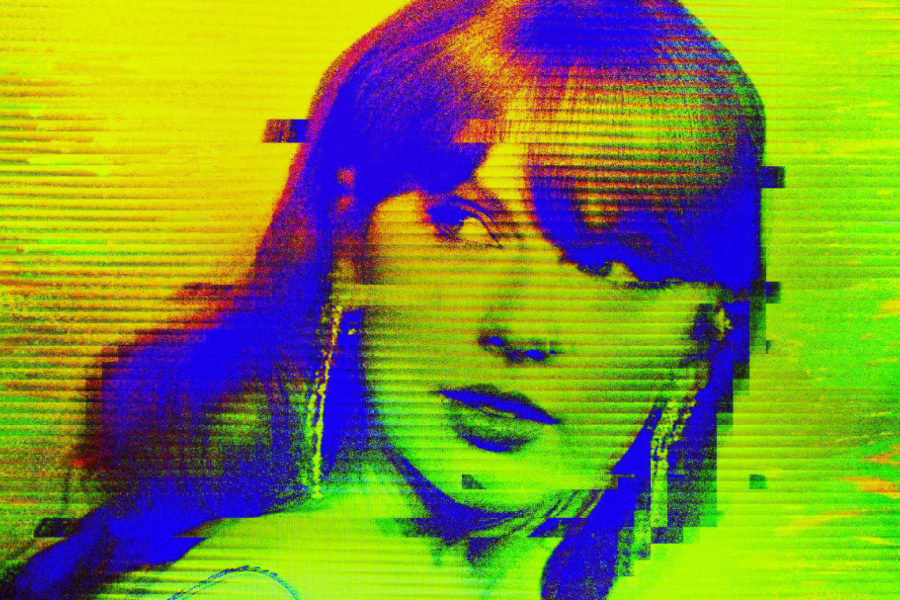
What Happened
Last week, AI deep fake pornographic images of Taylor Swift were shared broadly across X (formerly Twitter) spawning AI regulation and causing X to shut down all searches for Taylor Swift. Researchers at Graphika and 404 Media attributed the start to 4chan message boards where a ‘daily challenge’ asked users to create adult AI images with the best proprietary engine (DALL-E3 and Bing) instead of the more common open source models.
In the thread, users shared tips and the latest tricks for prompt evasion, fake accounts and a focus target of celebrities spawning a flurry of images that were well received in the community before being shared more broadly to X and other platforms where they were viewed millions of times.
Notably these deepfakes have caused responses across tech companies from X blocking the search of Taylor Swift to Microsoft tightening safety controls for some of the prompts jailbreaks used. And regulators are responding as well – looking to tighten AI policies.
This was just the start
Just days later, deep fake robo calls impersonating Joe Biden and separate deep fakes of Taylor Swift emerged supporting Trump in opposition to her previously stated views.
There have long been fears about the risk of deep fakes, but we are only now starting to see them used in real life with real impact. This is also becoming easier as bad actors discover and share new prompt jailbreaks.
The ‘How’
Board users challenge each other to event AI safety regulations for mainstream AI tools, but the more daily activity is using tools like stable diffusion tools + collabs, and training data sets prompts to train on prompt and fetish content. These “morphs” can be transformed into a likeness to celebrities, cartoons or real people. These are then altered into pornographic poses.
Some of the tooling bad actors have already developed are:
- Tips for setting up fake accounts
- Prompt guides
- Colabs for training stable diffusion models
- Adult Image datasets to train models
From our view, there are clear parallels to the cyber and fraud intelligence space. For LLMs prompt evasion, the attack surface is the amount of combinations of text you can fit in a context window. It’s hard to defend against every possibility using simple rules, keywords, and even model training. Similarly, as cyber practitioners know, the list of CVE’s can be endless, but gaining intelligence on what’s targeting your sector and what’s most likely to impact you can help you prioritize resources to mitigate risk.
How to limit abuse
As AI-system abuse continues to evolve, we see a clear value in a threat intel approach, including:
- OSINT Threat intelligence analysis to understand latest ways systems are exploited
- Red teaming of AI systems
- Safety AI bounties — bug bounty equivalents
- Leveraging anti abuse models to review original models
- Policy innovation and processes to determine what should be safe.
To echo what Christina Lopez said at Graphika “AI companies should get ahead of the problem, taking responsibility for outputs by paying attention to evolving tactics of toxic online communities reporting precisely how they're getting around safeguards.”